197 times faster than the standard attention!Meta launched a multi -headed mechanism "Nine -headed Snake"
Author:Quantum Time:2022.09.19
Greater Section from the Temple of Temple | Public Account QBITAI
Although Transformer has begun to "show his skills" on many visual tasks, there is another problem.
That is to calculate the calculation on a large image.
For example, when facing a 1080P map, it will cost more than 60%of the calculation amount on the creation and application attention matrix.
The reason is mainly because the number of self -attention heads is the square of Token, and the number of token has a secondary relationship with the size of the graphic.
What can I do?
The good news is--
Now Meta has a bull -headed operation method, which can achieve 197 times faster than standard attention!
And while increasing the calculation speed, it does not sacrifice accuracy, and sometimes even increases accuracy by 1-2 points.
What's going on?
Source of ideas a "contradictory point"
This method is called Hydra Attention, which is mainly for Vision Transformer.
("Hydra" has the meaning of "nine -headed snake", from Greek mythology.)
Hydra Attention 'ideas originated from a little contradictory point in linear attention:
Using the standard poly head, adding more heads to the model can maintain the calculation quantity unchanged.
After changing the operation order in linear attention, increasing more heads will actually reduce the calculation costs of the layer.
As a result, the author creates a linear attention module by setting the number of attention in the model into the number of feature to create a token and Feature.
Specifically:
When the standard self -attention head is the square (O (T2D)) of the number of token, we re -arrange the order of the operation by using the Decomposable Kernel to turn the number of attention heads into the square of feature D.
Then use Hydra Trick to maximize the number of attention head H, so that H = d can eventually turn into a simplified operation of O (TD) in space and time.
Among them, the basis of Hydra Trick is shown in the figure below:
The author trains the Deit-B model with different heads on ImageNet-BK, including using standard self-attention (blue line, SoftMax) and multi-headar attention (red line, based on the similarity of the string).
The former occurred in H> 96. The latter occurred at the time of H <3.
When they add more heads to the model, the accuracy of the SoftMax attention model seems to collapse, and the poly head -linear attention can still be consistent, so there is the above operation.
(It should be noted that H must be divided by d = 768.)
The speed is 197 times, and the accuracy rate can be more upper level
Let's take a look at the transcript surrendered by Hydra Attention.
It can be seen that Hydra's attention is 197 times faster than the standard (t = 197).
As the size of the image increases, it significantly increases the number of FLOPs of the model, and has always been only 0.02%in the calculation of the creation and application attention matrix.
In addition, the author found that using different kernels, Hydra Attention can also increase the accuracy of the model about two percentage points.
Or replace the specific attention layer with Hydra Attention, which can also increase the accuracy of the model by 1%or remain unchanged with the baseline.
Of course, up to 8 layers of replacement.
In addition, the author said that this method should be expanded to the NLP field, but they have not tried it yet.
about the author
This result has been selected into ECCV 2022 Workshop.
The author has a total of 5, from Meta AI and Georgia Institute of Technology.
Among them, 3 Chinese people are:
Cheng-Yang Fu, graduated from Tsinghua University, graduated from the University of North Carolina University Church Mountains, is now a research scientist in Meta computer vision.
Xiaoliang Dai, graduated from Peking University undergraduate, graduated from Princeton University, and worked at Meta.
Peizhao ZHANG, who graduated from Sun Yat -sen University, is a PhD at the University of Texas A & M. He has worked at Meta for five years.
- END -
Dangdang is the biggest hotbed of pirated book vendors?

Source: Bianws official accountOn July 6, after the Huawei Chen Chunhua incident, ...
After the market value evaporates 65 billion, the "mask king" is steadily medical treatment, and the condom
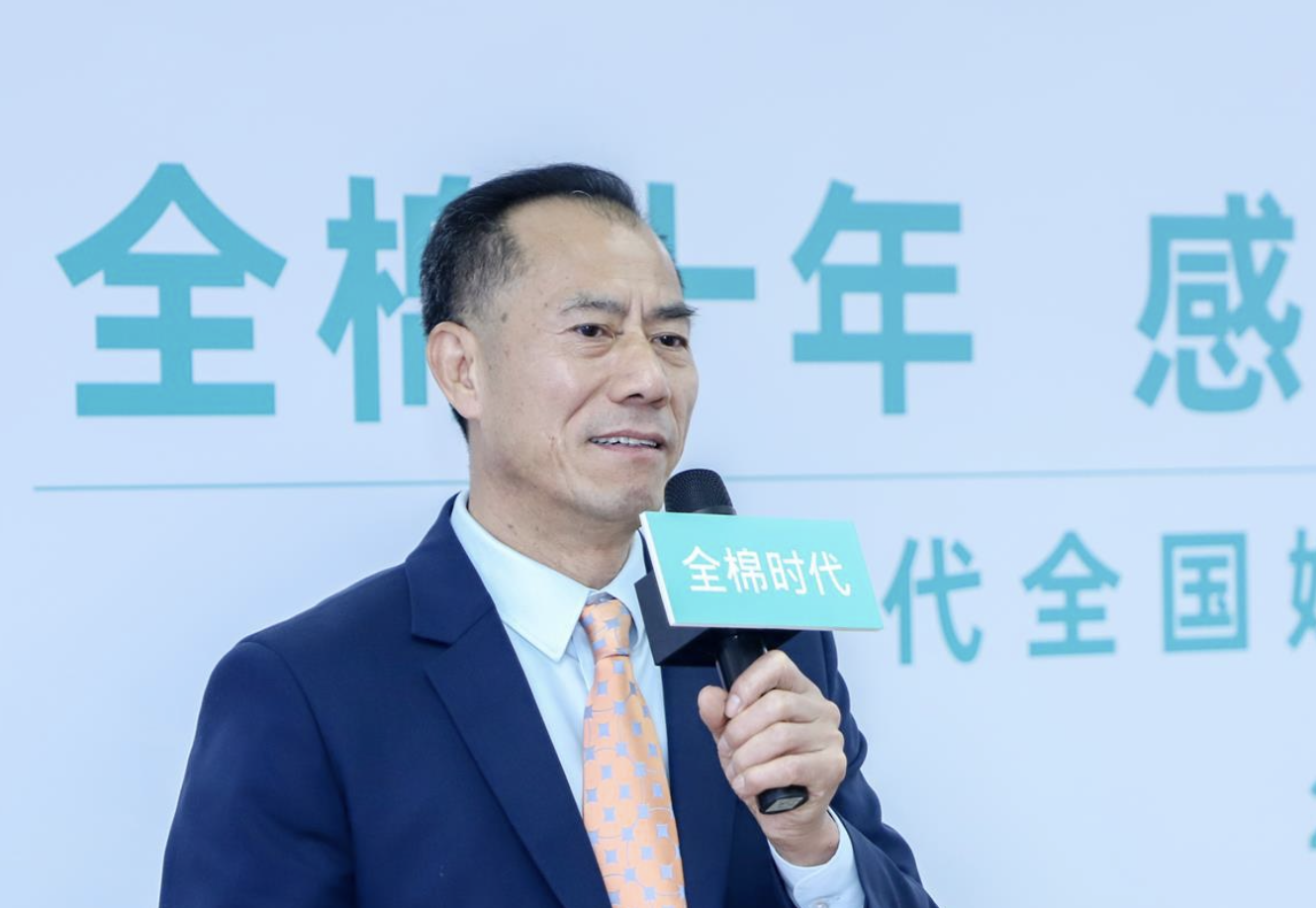
The decline in performance is a true portrayal of some medical device companies.Wh...