A NERF gets full -scale: Hong Kong -China University team BUNGEENERF can be rendered from architecture to the earth
Author:Quantum Time:2022.08.15
Pine sent from the quantity of the Temple of Temple | Public Account QBITAI
Do you dare to believe that this is the 3D scene rebuilt by AI?
Such a large -scale scene can still maintain such a high clarity. It is certain that it is not wrong with Google Maps?
not at all! This is a model proposed by the Hong Kong -China University team: BUNGEENERF (also known as Citynerf), which is suitable for the restoration of various scale scenes.
At present, this paper has been included by ECCV2022.
From a single building to the large -scale scenes such as the entire earth, you can restore it into 3D scenes through multiple 2D pictures, and the details are also in place.
Netizens are also very excited about the research results of BUNGEENERF.
This may be one of my favorite NERF projects in recent weeks. This is a very interesting and exciting result!
How does BUNGEENERF do so powerful?
Multi -level supervision and gradual model
Multi -scale restoration of 3D scenarios will cause large -scale data to change, which also means to increase learning difficulties and change the focus of images.
BUNGEENERF establishes and training models in a gradual way. It uses a gradual neural radiation field to represent a variety of scenarios under multiple scale. Photos used to generate 3D scenes include various perspectives and distances.
This gradual way is divided into the work of each network layer, and the position encoding can activate different frequency band channels at different standards and release the corresponding details at each scale.
It can not only render the details of large -scale scenes, but also maintain the details of the next scene.
Specifically, this model can well restore the 3D scenes under various standards to the following two parts:
The first is that it has a gradual growth model of the residual block structure, which can solve the problem of pseudohadoscopic shadows under the large scale of the previous model.
The total number (LMAX) of the BUNGEENERF model is preset first, and the number of times of this training is the number of paragraphs after the camera and the scene are separated continuously.
In other words, the training at all stages of the model refers to training under different scale.
Then start with the remote view (L = 1), and as the training is performed, the BUNGEENERF will be incorporated into a closer scale (L+1) during each training stage.
By allowing the model to invest more in the peripheral area in the early training phase to make up for the deviation of the sample distribution.
During the training stage, the growth of the training set accompanied by the increase in residual blocks.
Each residual block has its own output head, which can be used to predict color and density residues between continuous stages. When observing at close range, the new complex details appear in the scene.
Followed by BUNGEENERF with a tolerance multi -layer supervision structure.
Because the quality of the image rendering under all scale should be maintained, during the training phase, the output head is jointly supervised by a larger scale before. The loss at this stage will be summarized on the output of all the previous scale.
The design of the multi -level supervision has taken into account the complexity of the details at a deeper output head, so the rendering view will be clearer and more.
Compared with the details of the details of other models on various scale, the effect of BUNGEENERF is more obvious.
Full -scale detail rendering
The research team gives the comparison of 3D scenes generated by BUNGEENERF and other models generated by BUNGEENERF. Bundenerf is significantly better than other models and is close to the real scene.
In addition, BUNGEENERF allows to withdraw from different residual blocks to control the LOD (detail level).
When enlarging the image, the latter output head gradually adds more complicated geometric and texture details to the rough output of the first output, while maintaining the characteristics of the relatively shallow learning of the early output head.
If you are interested, you can poke the link to learn more ~
Reference link: [1] https://arxiv.org/pdf/2112.05504v2.pdf=] https://city-super.github.io/citynerf/= https://twitter.com/xingangp/ Status/1553014023871922176
- END -
Chaozhou Xiangqiao District: The first batch of 51 college students should take a medical examination
In order to do a good job in the physical examination of the recruitment and ensure that the selection of outstanding college students should be enlisted, on June 17, the Xiangqiao District Requesting
A registers of the Fifth and Sixth Army of the Japanese Invasion of China were made public for the first time!
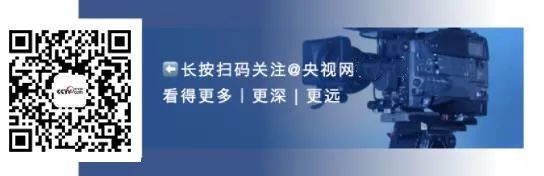
On the occasion of the 77th anniversary of the 77th anniversary of the 77th annive...