5000 words Explain corporate data security governance
Author:Data School Thu Time:2022.09.27
This article is about 4700 words. It is recommended to read 10+ minutes
The DGPC framework is constructed around 3 core capabilities, covering three parts: personnel, process and technology.
In 2010, in response to data security governance, Microsoft proposed a data security governance framework (DGPC) that specializes in privacy, confidentiality and compliance. It is hoped that enterprises and organizations can achieve their goals in a unified interdisciplinary manner, rather than different departments in the organization. Independent implementation. The DGPC framework can work with the company's existing IT management and control frameworks (such as COBIT), as well as ISO/IEC27001/27002 and Pay Card Industry Data Security Standards (PCI DSS). The DGPC framework is constructed around 3 core capabilities, covering three parts: personnel, process and technology.
The DGPC framework provides a data security governance framework with privacy, confidentiality, and compliance. It focuses on the data life cycle and core technology fields, but it is mainly based on the methodology level. Detailed explanation of data security governance measures in all aspects of the data life cycle.
By 2017, at the Safety and Risk Management Summit, analyst Marc delivered a speech entitled "2017 Data Security" and mentioned the "data security governance". MARC compares it as the "Eye of Storm" to describe the important position and role of data security governance (DSG) in the field of data security.
Gartner对数据安全治理的基本定义是:“数据安全治理绝不仅是一套用工具组合而成的产品级解决方案,而是从决策层到技术层,从管理制度到工具支撑,自上而下、 The complete chain that runs through the entire organizational structure. Each level of the organization needs to reach a consensus on the goals and purpose of data security governance to ensure that reasonable and appropriate measures are taken to protect information resources in the most effective way. "
So, how do you do it in China?
On August 30, 2019, the "Mature Model of Information Safety Technology Data Security Capability" (GB/T 37988-2019) is referred to as DSMM (DATA Security Maturity Model) official Essence DSMM is not only a set of standards, but also a set of methodologies. The idea of the maturity model (CMM) of the ability of the ability of the ability. The DSMM standard is data -centered, the entire life cycle and data of the data are safe. The level of personnel capabilities is determined to evaluate the level of data, thereby improving its own data security level.
How to carry out data security governance
1) Principles of data security governance
Before carrying out data security governance work, the principle of data security governance is clarified. These principles will run through all our subsequent data security governance work. There are four principles:
Driven compliance, fully understand all laws and regulations, industry supervision, and local policies, and meet the requirements of compliance, while taking into account the actual development of the business.
Taking data as the center is the core technical idea of data security work; it is the information system, operating environment, and business scenario involved in various links involved in the data security work of data security. And operators are supported by data security protection.
Taking organizations as a unit is the core management idea of data security governance; data will circulate in different servers, products, and services. Organizations with or using data are the main body of data security responsibility and the basic unit of data security governance.
Based on capacity maturity as the basic starting point, the level of data security capacity maturity of an organization shows the comprehensive ability level of this organization in data security protection.
2) Construction ideas for data security governance
Let's introduce the construction ideas of data security governance. We start from the level of organization, system, technology, and operation, and establish a sound data security management system around the data life cycle. Organization and systems are the prerequisite for enterprises to carry out data governance. Technology and operation systems are the means to implement data security governance.
The organizational structure is divided into four layers, including the decision -making layer (data security leadership group), management (data security management team), executive layer (data security execution team), and supervision layer (data security supervision team).
With the organizational structure, we must also formulate a perfect institutional process. The institutional process is an important prerequisite for ensuring the implementation of data security governance. For example, the management system, operation specifications, workflow, auditing mechanism, etc.
With the organization and system, the corresponding technical tools that can be gradually landed next. For example, sensitive data identification, classification classification tools, decryption/desensitization, identity authentication, permissions control, operation audit, etc.
In the process of building an organizational structure, institutional process, and technical tools, a comprehensive operating system is gradually formed to comprehensively improve the data security operation capabilities. At the same time, the entire construction ideas must be driven by compliance and effectively carried out the data life cycle.
3) Implementation process of data security governance
With the idea of construction, we can implement the work next. We usually divide the implementation process into seven steps:
STEP1 Enterprise Status Research: Including corporate structure, network topology, security management status, business processes, data processes, etc. STEP2 data asset combing: Data assets are issued through business surveys and automatic scanning, data classification and classification standards are formulated, and data assets are sorted out and marked.
STEP3 security risk assessment: Risk assessment around the data life cycle, analyzing the benchmarking analysis according to various laws and regulations.
STEP4 Security System Design: Based on risk assessment, organizational structure, business process, data process, design data security management and technical system, and establish a management system.
STEP5 Technical Tools Implementation: Based on classification and classification, risk assessment results, construction data security technical tools, sensitive data recognition, desensitization encryption tools, access control, log audit, etc.
STEP6 Quick Pilot Verification: Through pilot operation, timely finding possible management vulnerabilities and technical defects, and discovering possible insufficient operations in time through regular audit.
STEP7 continues to optimize improvement: set up operating indicators, regular audits, continuous optimization improvements, nurturing management, technology, and operating systems, and continuously improved data security water levels.
Research, sorting and evaluation are carried out from top to bottom, and it is in line with the Gartner "data security governance" introduced earlier, and it is also a systematic design that completes the implementation of corporate data security.
4) Technical system for data security governance
The work of the entire data security implementation process is to implement the technical tools. The data security technology system can be divided into four parts: risk recognition, security defense, security monitoring, and safety disposal. Around the entire data life cycle.
5) The technology implementation route of data security governance
The technical system of data security governance is relatively complicated, so the implementation of data security governance is not achieved overnight, and it can be divided into three stages to complete:
In the first stage, the risk recognition of data assets and data assets was mainly identified. The classification and grading of data assets was achieved through sensitive data recognition, and security risk verifications were conducted for different grading data.
In the second stage, the construction of security capabilities in various scenarios of data applications has initially realized the data security, comprehensive and controllable, and controlled data security risk perception of data security risks, and improved internal control security guarantees. It mainly includes analysis of users and physical behavior, application gateway construction, API gateway construction, data desensitization, and watermark traceability. Realize all -round protection of key security scenarios through technical tools.
In the third stage, the data security construction is fully completed, and the coverage system is covered to various scenarios of data applications. In the data security use link, related data security technology can guarantee the security of data, and all operations have audit and protection measures. Through the improvement of data security risk perception, the global visibility of data security risks is achieved, and the comprehensive protection capabilities of identification, understanding, analysis and response of data security threats have been greatly improved from a global perspective.
It is worth mentioning that in the entire technology implementation route, there are two points that need to be grasped well, one is the choice of data security governance entry point, and the other is the focus of data security governance and difficulties.
Data security governance entry point -data classification classification
In September 2021, the "Data Security Law" specifically established the "data classification classification protection system" and its basic principles.
Data classification has become the basic work of establishing a unified and perfect data life cycle security protection framework. It can help enterprises make comprehensive inventory of data assets, understand sensitive data distribution, types, and order of order, so as to make a number of enterprise -level data asset directory, and lay the foundation for future enterprise data asset management and data security system construction. Essence At the same time, different security measures are adopted for different classification data, and data protection and data circulation are balanced to maximize data value.
So, what should I do for the data classification classification?
The functional architecture of the data classification hierarchical platform is mainly divided into four modules, namely data assets automatic discovery, data intelligent analysis engine, sensitive data recognition algorithm library, and classification hierarchical panorama.
The first module is the automatic discovery of data assets. Through automatic scanning data assets, and at the same time, data assets are sorted out and marked, and a set of data asset lists are finally formed to lay a good foundation for enterprise data asset management and data security system construction.
The second module is the data intelligent analysis engine. Data classification classification is actually a process of data analysis. After the data asset list, data content analysis (NLP /corpus), strategic rules (regular expression /keywords) and machine learning models can be used Intelligent analysis forms a set of data classification strategic rules and model templates.
The third module is the sensitive data recognition algorithm library. Built -in sensitive data intelligent identification algorithm library, covering common high -sensitivity personal information and business information, such as names, gender, mobile phone, ID card, etc., helping enterprises automate and efficiently identify sensitive data, and sort out sensitive data assets.
The fourth module is the data classification hierarchical panorama. Automation periodic scanning data assets, intelligent classification classification, identifying sensitive data, generating data classification and hierarchical panoramic diagrams, and supporting the diversified output method of classification and classification results.
Taking the data classification classification practice of a bank as an example, based on the intelligent classification grading platform independently developed by Jidun Technology -Jidun · Smart Differential, and in -depth understanding of the customer's business needs, finally completed: 1. Sorted the 100,000+ fields, and the 100,000+ fields were sorted out. Forms 5000+ strategy rules, divided into 5 sensitive levels (extremely sensitive, sensitive, sensitive, low -sensitive, and unsightly).
2. Four layers of data formation, including parties, products, agreements, time, accounts, media, channels, resources, resources, and general -purpose 9 major classifications.
3. The total number of sensitive information recognition exceeds 10000+, the accuracy of identification is 100%, and a sensitive information management mechanism and the standardized operation specifications have been established.
After completing the data classification classification, the next step is to formulate a data classification classification protection strategy, that is, different security protection measures for different classifications of different levels of different levels. First, you need to clarify the data security control architecture, then sort out the data application scenarios, and finally formulate data classification and classification protection strategies.
Data security governance is difficult -the use of data use security
Due to the great liquidity of the data use link, the complexity of the application system scenarios, and the incomplete personnel control of the personnel, the pain points of data security construction are often concentrated in data use and sharing security.
Combining many years of data security practice experience, we creatively put forward data use security methodology: based on people as the core, based on business scenarios, based on data classification and classification, based on zero -trust framework and user and physical behavior analysis as the starting point, facing internal applications Data uses a full process to build a active security prevention and control system for data.
1. People as the core: During the use of system data, personnel are the subject of behavior, and through information, environmental information, environmental information, and relatively "static" personnel authority, organizational structure, job departments, etc. Main portrait, recognize the risk of personnel.
2. Focusing on business scenarios: Through the excavation of different dimensions such as accounts, permissions, access behavior, and data operations, identify an abnormal data to use access risks, and achieve accurate positioning judgment.
3. Based on data classification classification: Based on the introduction of data security gateways, based on sensitive identification and classification grading rules in the process of data access, identify the importance and sensitivity of the current access data, so as to carry out targeted protection.
4. Based on zero -trust framework: The entire zero -trust framework is mainly divided into two planes, data plane and control plane. In the data plane, collecting multi -party data, including access subjects and access objects, and then collected the data to the control plane, and the data analysis is performed through the strategic model to complete the dynamic alarm and automated response.
5. Users and physical behavior analysis are the starting point: based on zero -trust framework and artificial intelligence model behavior analysis technology, efficiently identify the behavior risk of data use, and conduct real -time response alarm. When necessary, link related business systems Block and interception.
The realization of all this requires a complete set of data to use the support of security control platforms. We divide this platform into four levels. They are data collection, data combing, security analysis protection, and safe operations.
Data security governance challenges and outlook
In view of the current overall data security external environment, as well as security incidents such as ransom and data leaks; the current and in the next few years will face more severe data security challenges. The brand reputation due to data security issues and events will be damaged, direct, and direct, and direct Indirect financial losses, external business interruptions, legal consequences, and regulatory agency notification punishment, sensitive data information leakage, etc. are unacceptable.
In 2022, the global network security threat entered the era of highly uncertain "Black Swan". Enterprise security and risk management faced unprecedented challenges. The main reasons were:
Lack of unified guidelines for enterprises
The national standards related to data security technology are currently introduced, and it is not yet effective to form an enterprise. For example, data encryption and desensitization have not yet formed a unified standard, and there are deviations in the understanding of each enterprise.
Data security talent lack
Data security well spraying demand has exploded, and data security risks in various fields need to be resolved urgently. The lack of data security talents in the industry in the industry.
Safety technology needs to be practiced
Traditional network security technology cannot meet the data security requirements. The data itself has the characteristics of sharing, replication, and fast circulation. Some technologies and solutions are in the research and development stage, and lack of application practice.
Complex business scenarios
The business is preceded by security, the business form has been solidified, many enterprises face data security transformation problems, the transformation is difficult, and the differences between various enterprises are different. The data security governance has a diversified business scenario's complex data security governance challenge.
Facing the challenge, we can sum up some clearer development trends:
National laws and regulations and standard systems are becoming more and more improved;
Data security law enforcement has gradually increased;
Data security governance moves from compliance drivers to self -driving;
Establish a continuous iterative data security governance system;
Improve the level of data security assessment/review automation;
The gradual development and application of emerging technologies continue to stimulate new needs for data security.
Edit: Huang Jiyan
- END -
The glowing phenomenon you have never seen, there is only one high -paste photo mysteriously
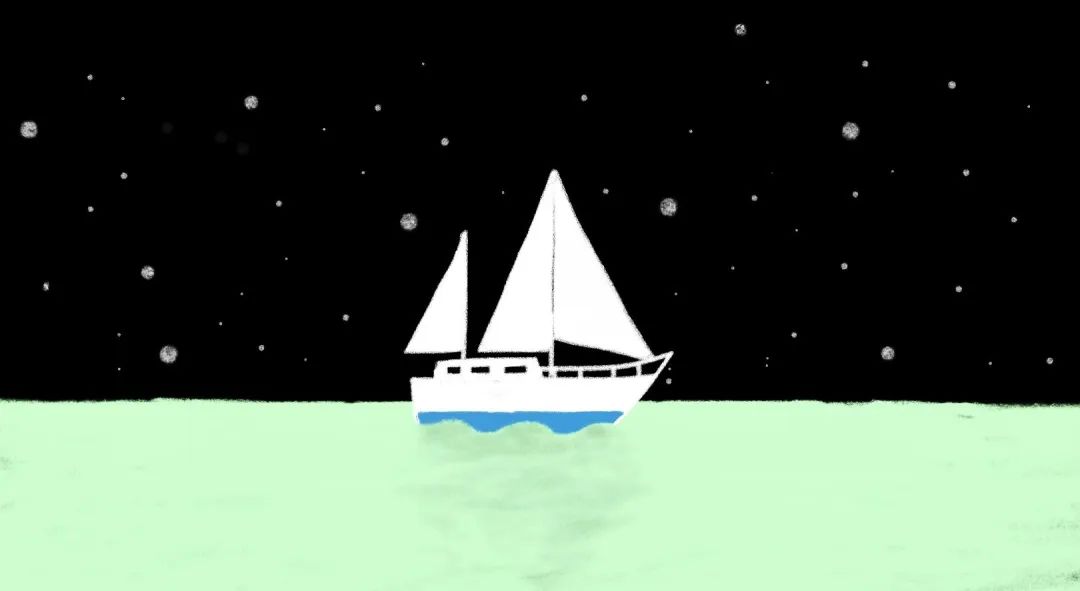
On the night of August 2, 2019, the private yacht Elephant God sailed into a glowi...
Quantum algorithm conquered a new problem

Source: scienceaiThis article is about 2100 words, it is recommended to read for 9...